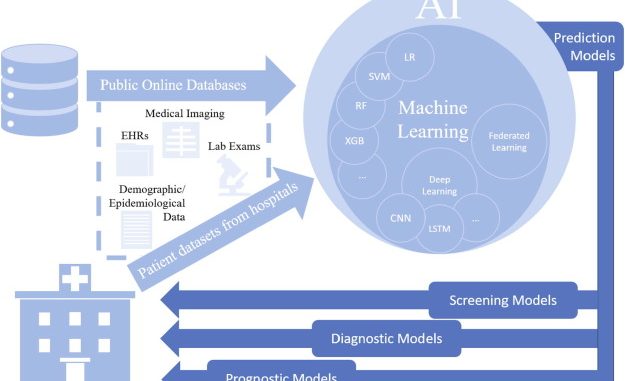
in which the authors express their thoughtful opinion about several simultaneous works by independent research groups worldwide (eg, Massachusetts Institute of Technology, National Research Council of Canada, University of Cambridge, and Swiss Federal Institute of Technology Lausanne). One of these works was our own; a pioneering, multicentre, international study
with a clinically validated dataset of forced coughs alongside quantitative RT-PCR from participants who physically attended a test centre. Participant control was performed on site by health personnel at the partner health centres that contributed to this study.
focuses on human audio biometrics in general, albeit eight out of ten referenced works made use of coughs as their audio source. The use of cough sounds to detect respiratory system abnormalities had been investigated for at least 3 years before the COVID-19 pandemic.
Cough analysis is a particular form of audio biometrics, and other forms of audio biometrics cannot be discussed interchangeably.
,
Subsequent research initiatives from public bodies must now help to enable inclusive research clusters and secure collaborative infrastructures in the domain of audio biometrics.
However, a general assumption is that a competent biometrics classifier should maximise the distinction between divergent patterns within participants, while minimising that of different participants within the same class. The inclusion of divergent observations (negative and positive) from the same participant, in the training set only, could help to satisfy this assumption; however, this effect requires further study.